AI in Higher Education Webinar Series
2: Research
1 April 2025
Here are the slides from James Ransom’s presentation at the event on AI in teaching & learning, organised by the National Centre for Entrepreneurship in Education (NCEE). Links are included for key texts. Contact details are at the end.
Thank you and welcome everyone. I warn you now this is going to be pretty speculative in parts. I will talk for around 35m about how I see things going. Counter arguments are welcome! Please post your reflections, experiences and comments in the chat as I go through.
Key takeaway
Universities should prepare for the possibility that the way research is conducted is going to undergo a seismic shift.
We’ll cover in this session what this means.
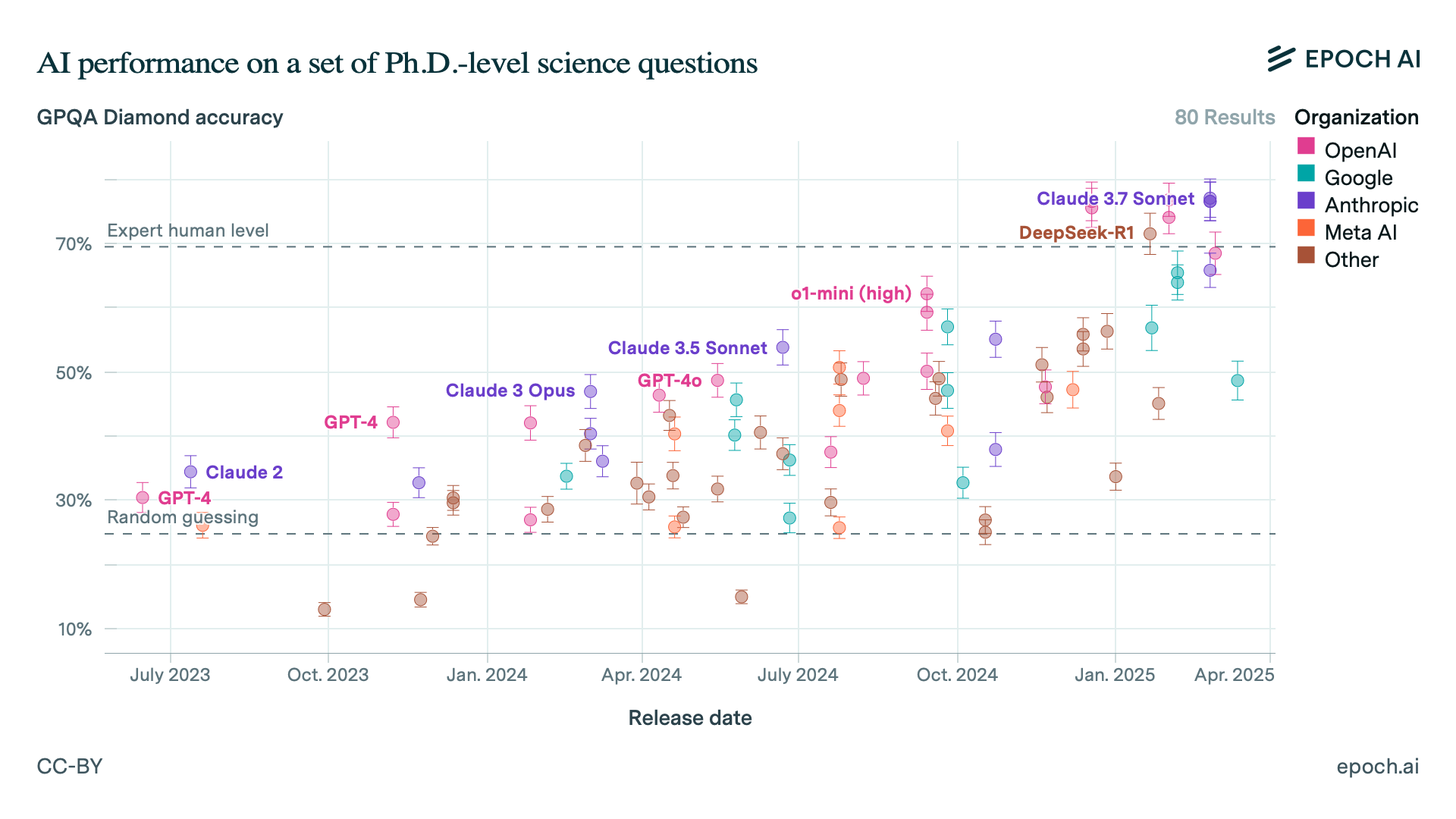
Let’s talk a little bit about the pace of change. This image was where we finished last time. Take note of the two dotted lines. The one at the bottom is random guessing, and the other higher up one is PhD level knowledge. We can see in the past few months that the latest so-called ‘frontier models’ have passed PhD level knowledge. This basically compares the ability of the AI model against a specialist PhD with access to the internet. Note that this is for science questions.
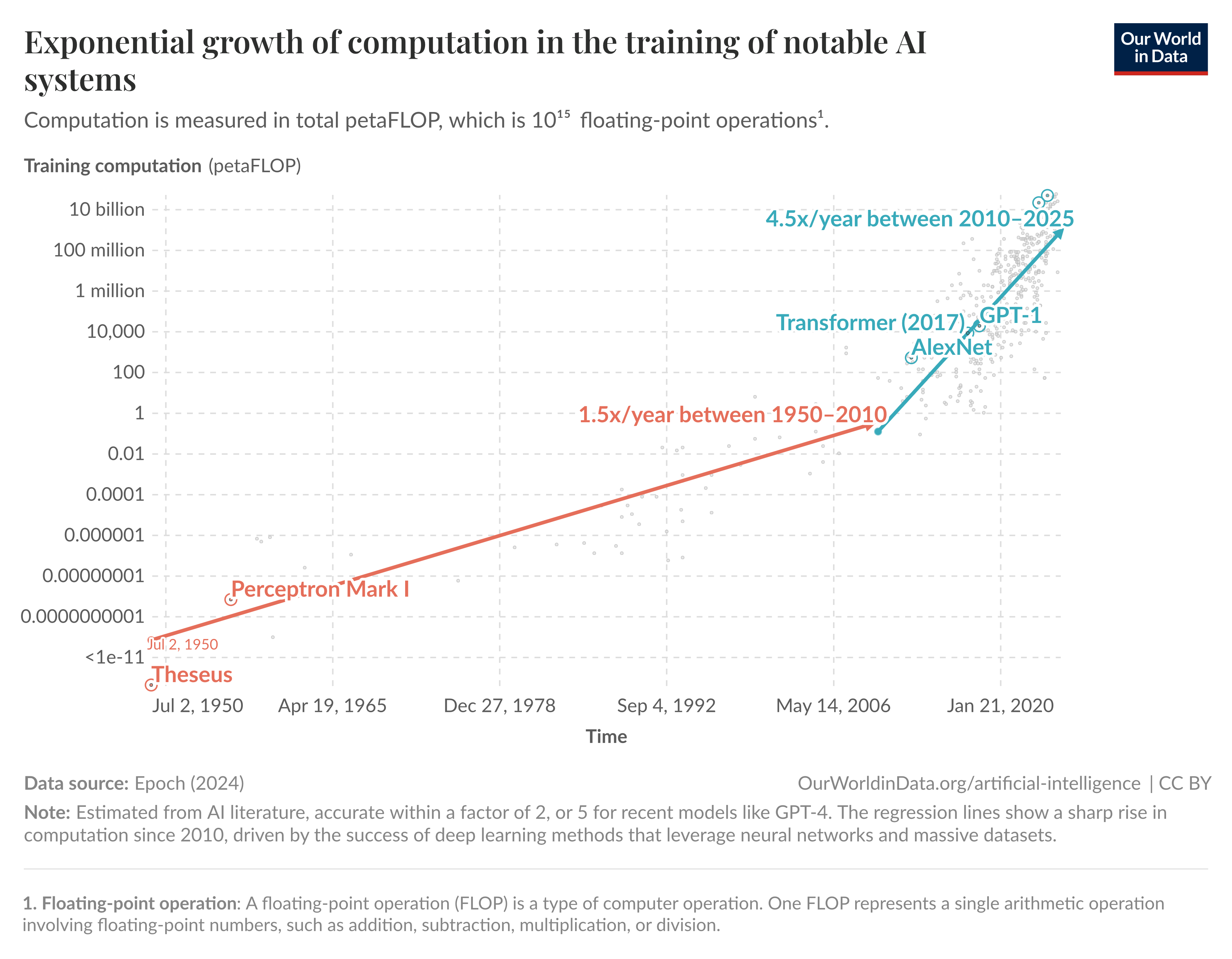
Here’s another one that shows the available compute over time and we can see it also rises exponentially towards the end. This shape of graph is pretty familiar when we look at AI development. We’ll see similar graphs throughout this presentation.
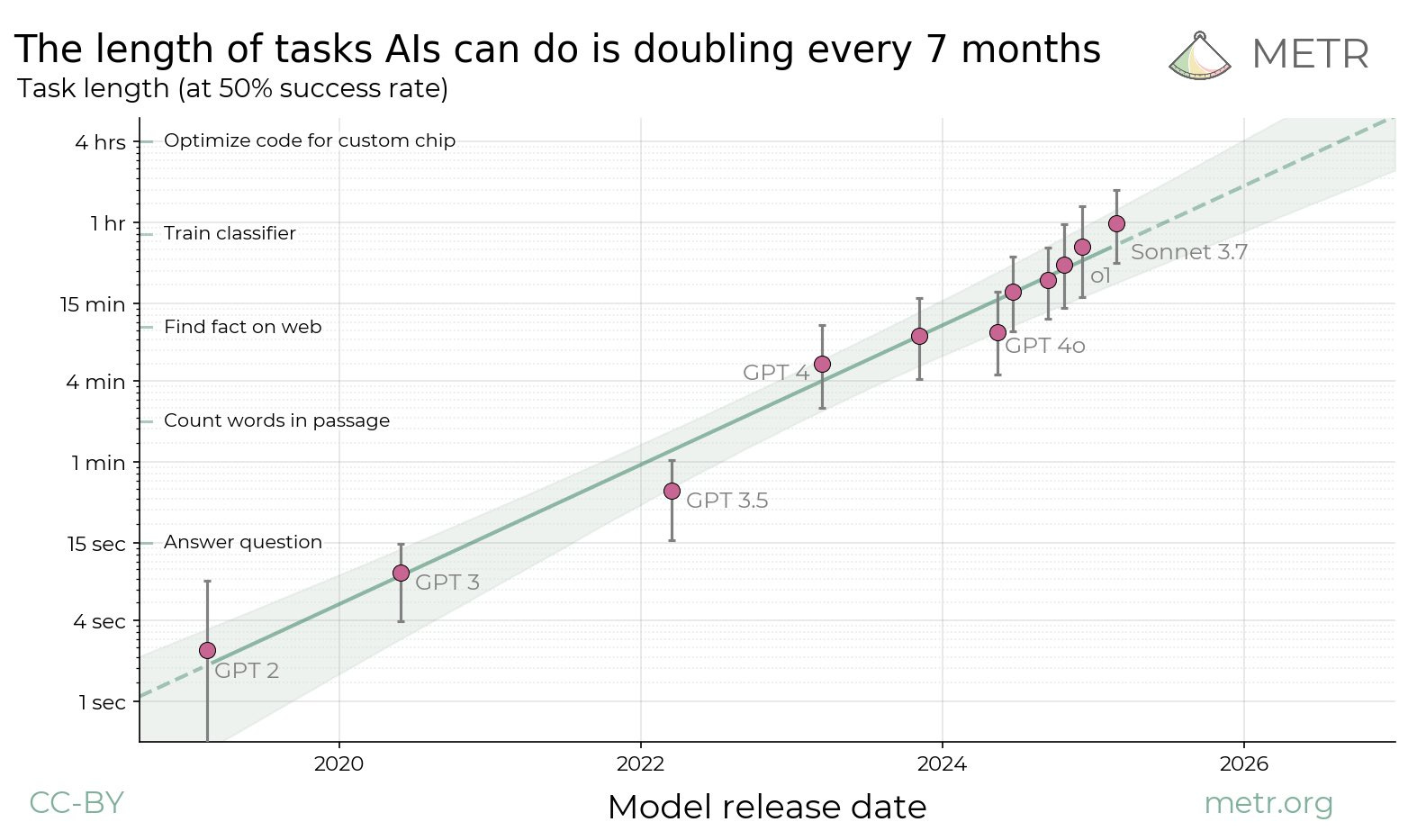
And here’s one showing that the length of tasks AIs can do is doubling every 7 months.
"Person after person – from artificial intelligence labs, from government – has been coming to me saying: It’s really about to happen… we are on a path to creating transformational artificial intelligence capable of doing basically anything a human being could do behind a computer – but better…
If graphs are not your thing, let’s consider some of the views of people who don’t work for the labs where this work is being done, but are quite well informed. This is US commentator Ezra Klein.
…they thought it would take somewhere from five to 15 years to develop. But now they believe it’s coming in two to three years, during Donald Trump’s second term."
He adds…
"I believe that very soon — probably in 2026 or 2027, but possibly as soon as this year — one or more A.I. companies will claim they’ve created an artificial general intelligence…
And here is Kevin Roose, technology columnist for the New York Times, a couple of weeks ago.
…I believe that most people and institutions are totally unprepared for the A.I. systems that exist today, let alone more powerful ones, and that there is no realistic plan at any level of government to mitigate the risks or capture the benefits of these systems."
He adds…
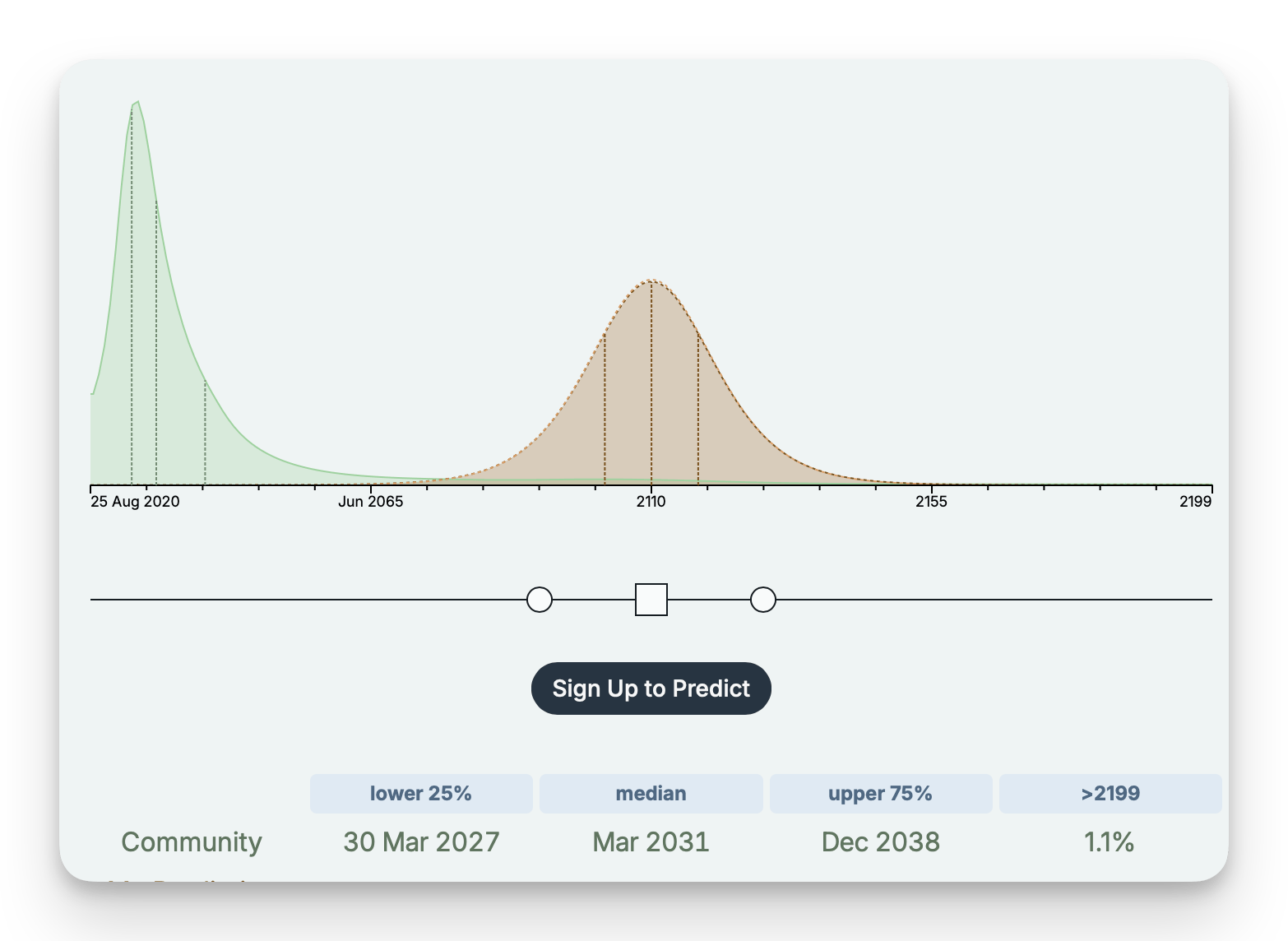
If the views of commentators also don’t do much for you, how about the aggregated views of people with a proven track record in making accurate forecasts about the future.
They forecast a 25% probability to AGI arriving within 1–2 years, and 50% for 2–5 years.
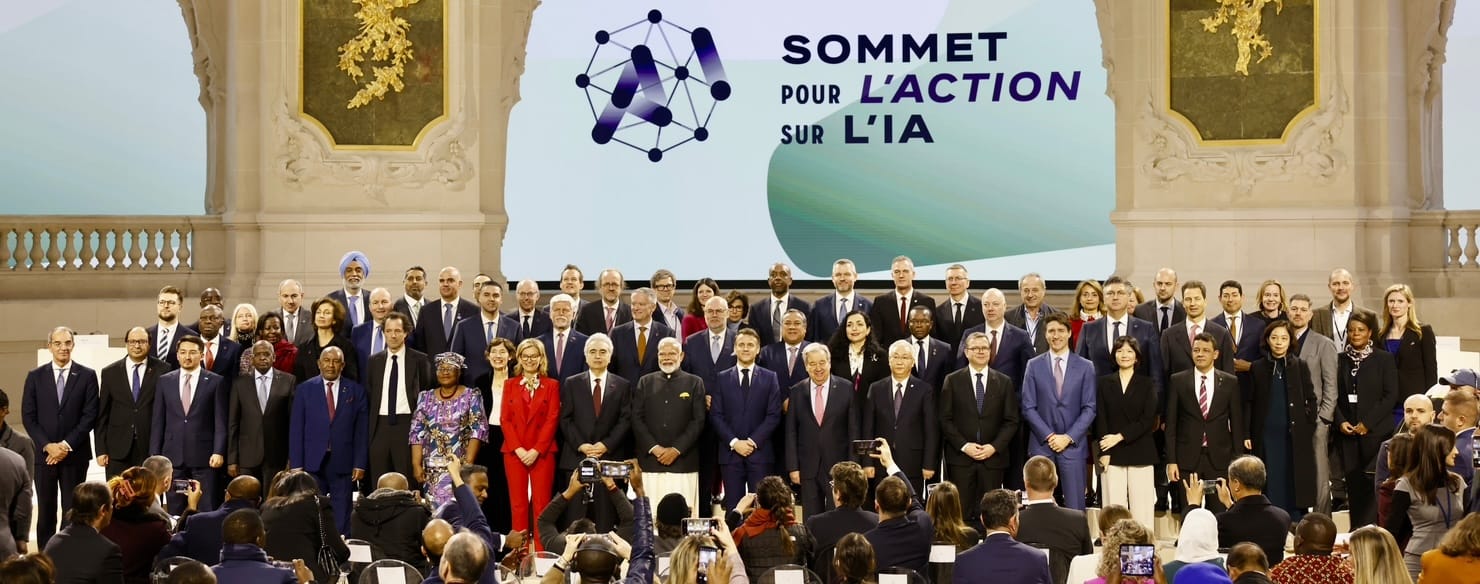
Or if none of this is very persuasive, we should take note of the fact that a conference on AI in Paris in February managed to attract Presidents, Vice Premiers, Vice Presidents.
“If ChatGPT is an amoeba, how do you think an AI T. Rex would look like?”
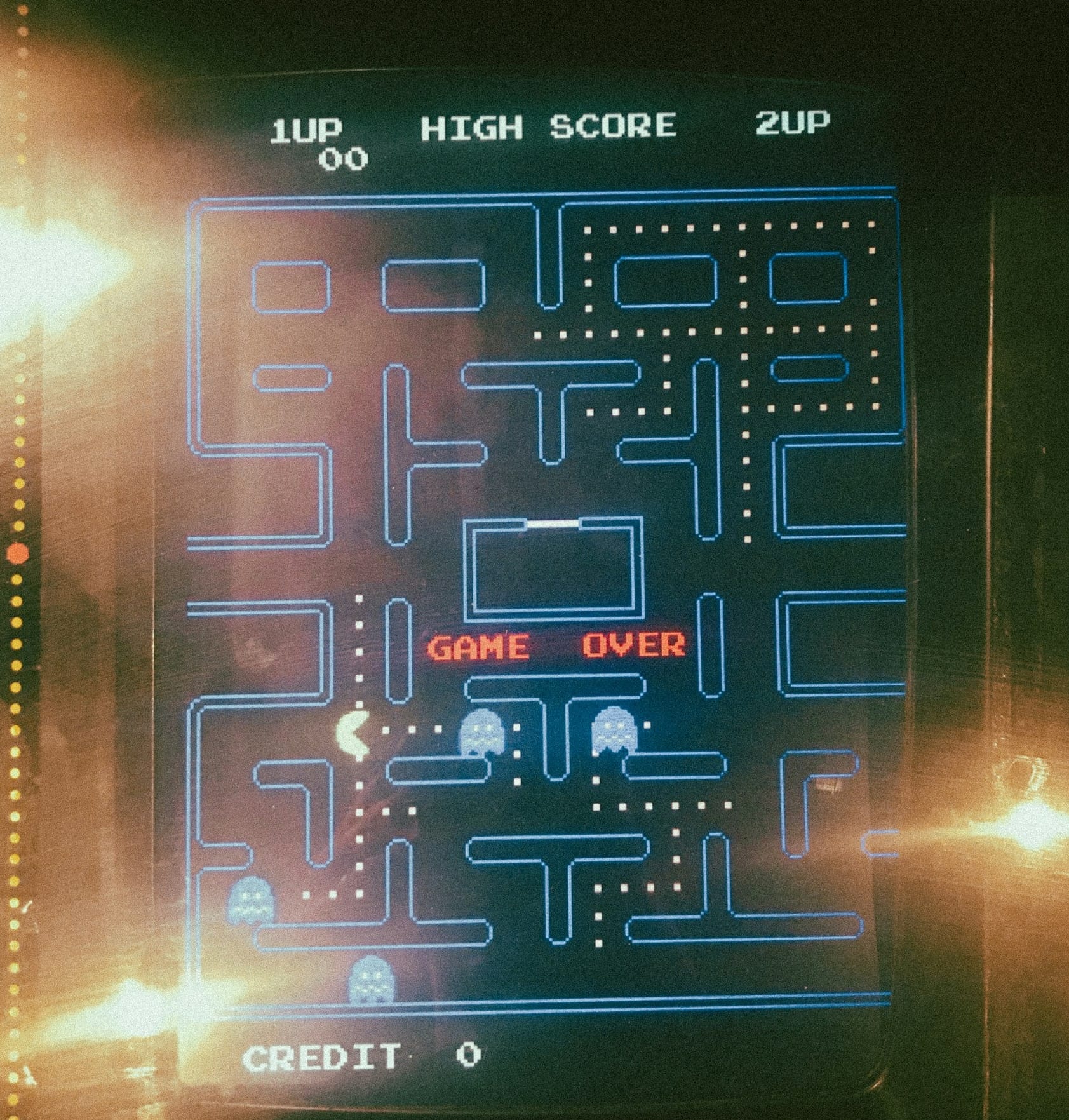
But it’s fair enough if numbers and predictions and politicians are not enough to signal that something might be happening. If this all becomes somewhat meaningless, there’s nothing better than an analogy. One of my favourites is from Yuval Noah Harari, author of the book Sapiens, who says “if ChatGPT is an amoeba, how do you think an AI T. Rex would look like?”.
Or there’s also a good one by Ethan Mollick who is a professor at Wharton in the US. In his book he says the AI you use today is the worst AI you’ll ever use. If today’s AI is Pac-Man, the future AI will be a PlayStation 6.
Key caveat
Past performance is no guarantee of future results
But, as they say, past returns are no guarantee of future performance. There are people, very bright people, experts in the field, who push back on all of these predictions. Achieving artificial general intelligence is going to be very, very difficult. It may not happen for a long time.
But what if we were going to see an intelligence explosion, which could be the result of getting closer to artificial general intelligence? What if none of this does happen, but we get closer, so somewhere between where we are now, and what some of these predictions suggest? What would that mean for universities?
There’s a chance it won’t happen, but universities still need to be thinking about what it could mean for them. And this is what we’re going to be covering today.
Dr James Ransom
About me
To introduce myself, for those of you who don’t know me, I am James Ransom, I am Head of Research at NCEE. I’m also a Senior Visiting Fellow at UCL Institute of Education.
The other thing that I would stress is that I am not an expert on AI. Although I’ve been doing work in this space my background is primarily on the role of universities in tackling societal challenges. But – and we’ll begin to see today, and even more so in the next webinar – these issues are actually quite closely linked.
Session | Time period | Scope |
---|---|---|
1: teaching and learning (previous) | Now | Narrow |
2: research (today) | ~1–5 years | Broader |
3: engagement with society (1 May, 12:00 GMT) | ~5+ years | Very wide |
So this is the second in a series of three webinars. Each one of the three seminars will have a focus on one of the core university functions.
The previous session two weeks ago focused on teaching and learning. The slides are available on our website, and these ones will be added as well.
Today’s session on research is looking one to five years ahead and is slightly more broad and speculative in scope.
And the final session will be the role of universities in society and how society will shape the role of universities. It will look five or so years ahead and has the broadest and most speculative scope of all.
So let’s dive in.
Where we just were
For the rest of this session we’ll travel in a time machine. We’ll begin with where we just were. We’ll then zoom forwards a little bit to where we are now and then we’ll continue to where we might be in 24 months. And we’ll finish up with where we might be in five or so years.
A note on terminology
Science vs. research; scientists vs. researchers
Let me add a note of caution to say that in a lot of the literature people talk about science and research interchangeably. And much of the discussion of scientists and science is focused on technological progress.
Some of the studies are specifically focused more on STEM subjects, some are more general. Some findings are likely to apply to some extent to other disciplines too, but in all of the discussions that follow there’s likely to be big variation across disciplines, and between different arts, humanities, social science, STEM subjects and so on.
So where were we just now? We need to talk about the state of knowledge production and there’s two key things going on here.
Ideas are getting harder to find…
The first is that research productivity is declining sharply - we now require 18x more researchers than in the early 1970s to maintain the same pace of progress.
Quite sophisticated studies have shown that papers and patents are increasingly less likely to break with the past or push science in new directions.
Another example: drug development costs double approximately every nine years, demonstrating increasing difficulty in biomedical innovation.
…this is despite an increase in research intensity
We have been exponentially increasing our research efforts.
Research effort in the US is over 20x higher today than in the 1930s, and the global number of scientists doubles every couple of decades - 75% of all scientists who have ever lived are alive today.
Academic papers are increasing by 4% annually, while citations are doubling every 12 years. The vastness of these publication fields is making it more difficult for scholars to get published, get read and get cited, and to have any form of meaningful contribution.
It also makes it harder to find novel connections between existing knowledge, which is an essential driver of innovation.
But populations are shrinking and we’ve picked most of the low hanging research fruit. Humans alone simply can’t sustain an increase in research intensity.
Where we are now
That’s the recent history: research is getting harder despite massive and unsustainable increases in intensity. So where are we now in terms of AI and research?
Less of a focus today is the clandestine use of AI for writing. We covered quite a bit about the writing process in the previous session.
But I will mention that there is plenty of evidence to suggest that AI is being used more and more to write journal articles and there are some blatant and unethical uses of AI that have somehow passed through peer review and proofreading and have ended up in journals. This is perhaps more a reflection on the publishers, in this case Elsevier.
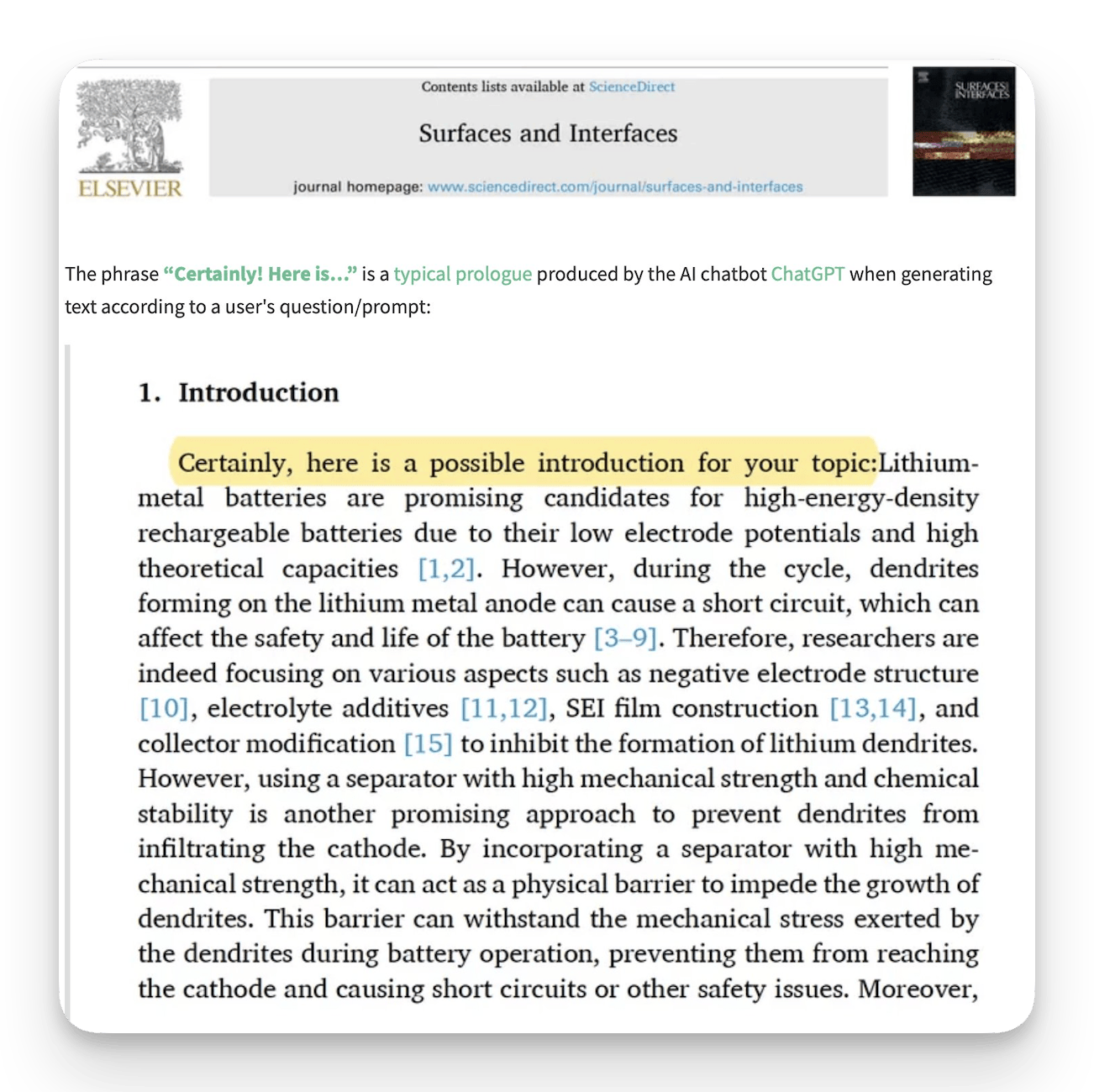
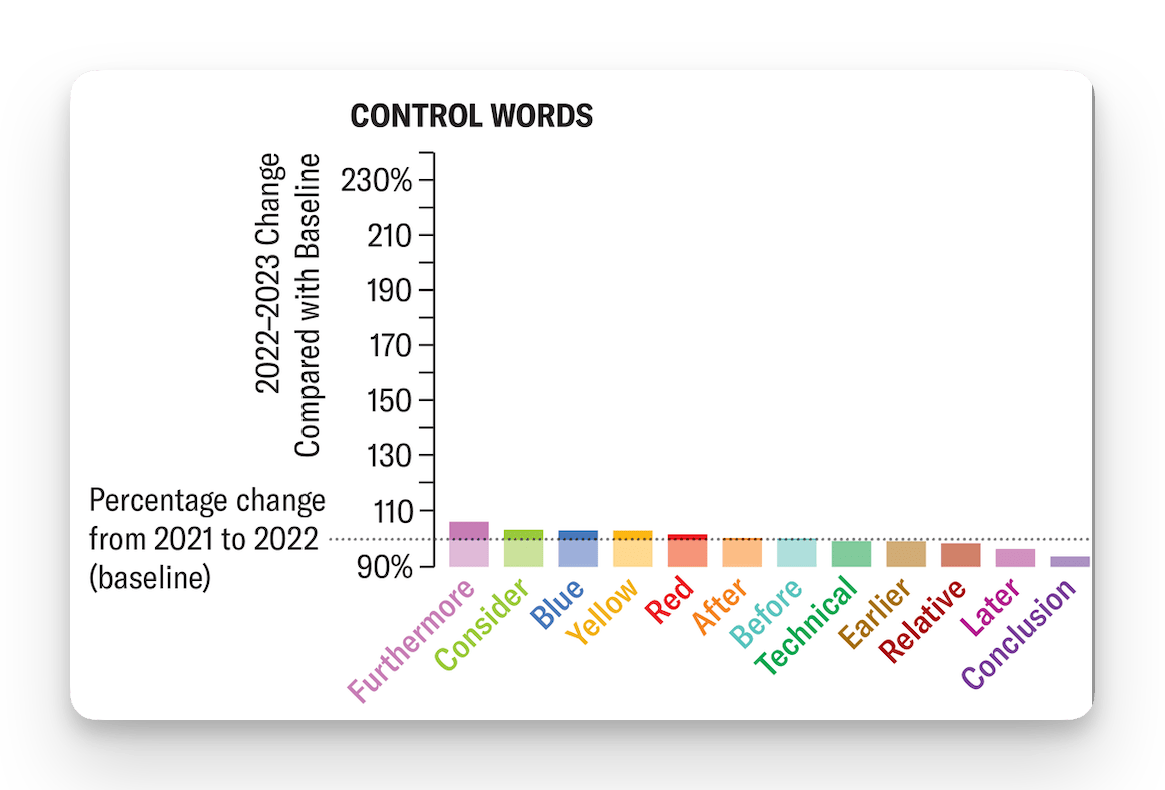
There have also been studies that have shown greater use of AI even without looking for bits of text that have been left in by mistake. So this study has a set of control words which appear consistently over time…
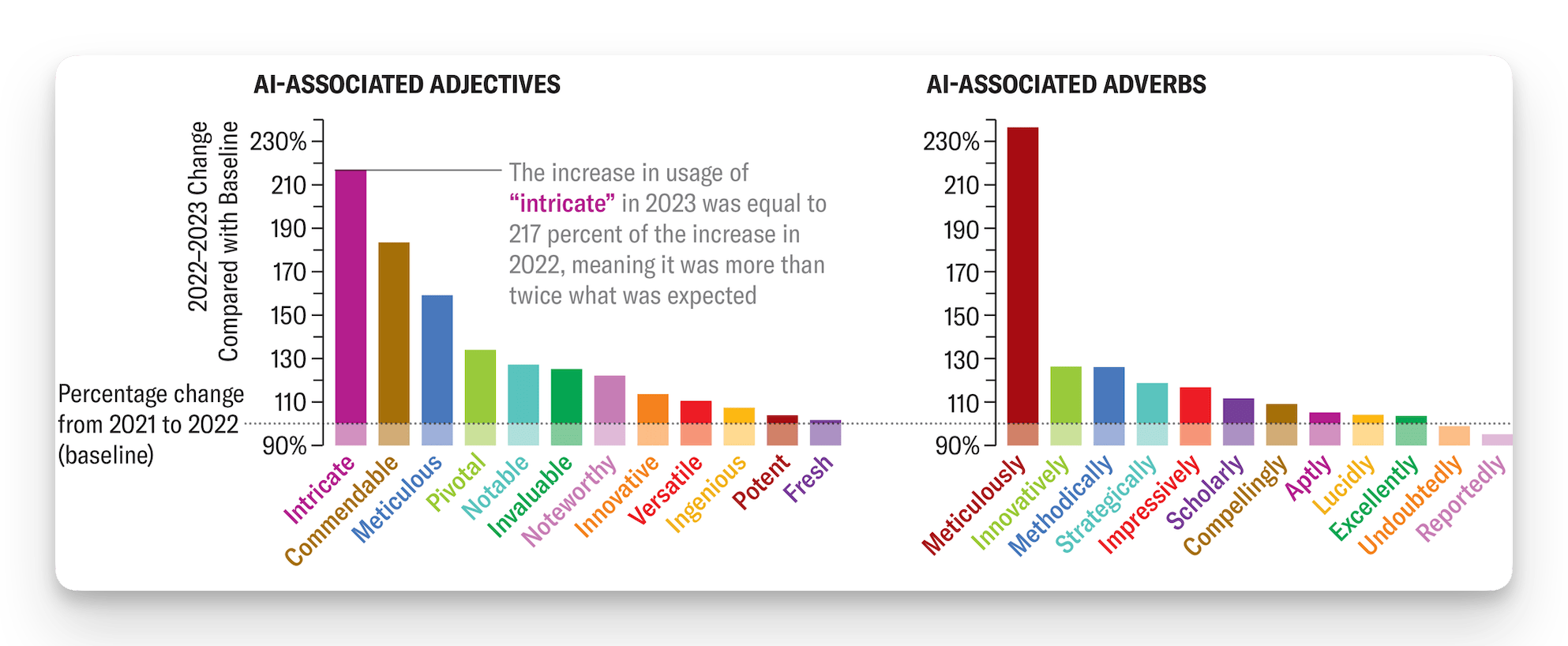
…and then a sharp spike in words that are commonly used by large language models in recent years. This particular study estimates that around 1% of all peer reviewed journal articles used AI as part of the writing process but this is already over a year or two old so it’s likely to have skyrocketed since.
There are also studies that have shown greater use of AI for peer review – again secretively and without declaring it.
AI can certainly be useful for writing, particularly for those who speak English as a second language. It may be helpful to speed up the very slow publishing process and it can be very effective at peer review if the assessment criteria is robust.
It is also possible to simulate REF exercises – for those of you who are not based in the UK, the REF is an assessment process of the quality of research which is used to determine research funding at UK universities.
But in all of these cases the use of AI needs to be declared and transparent. Otherwise we end up with AI writing articles, AI reviewing articles and AI summarising articles without humans really being involved and without the human reader knowing that this is the case.
Instead of this secretive use of AI, I’m more interested today in how it’s going to be used openly and deliberately to augment and perhaps replace human researchers.
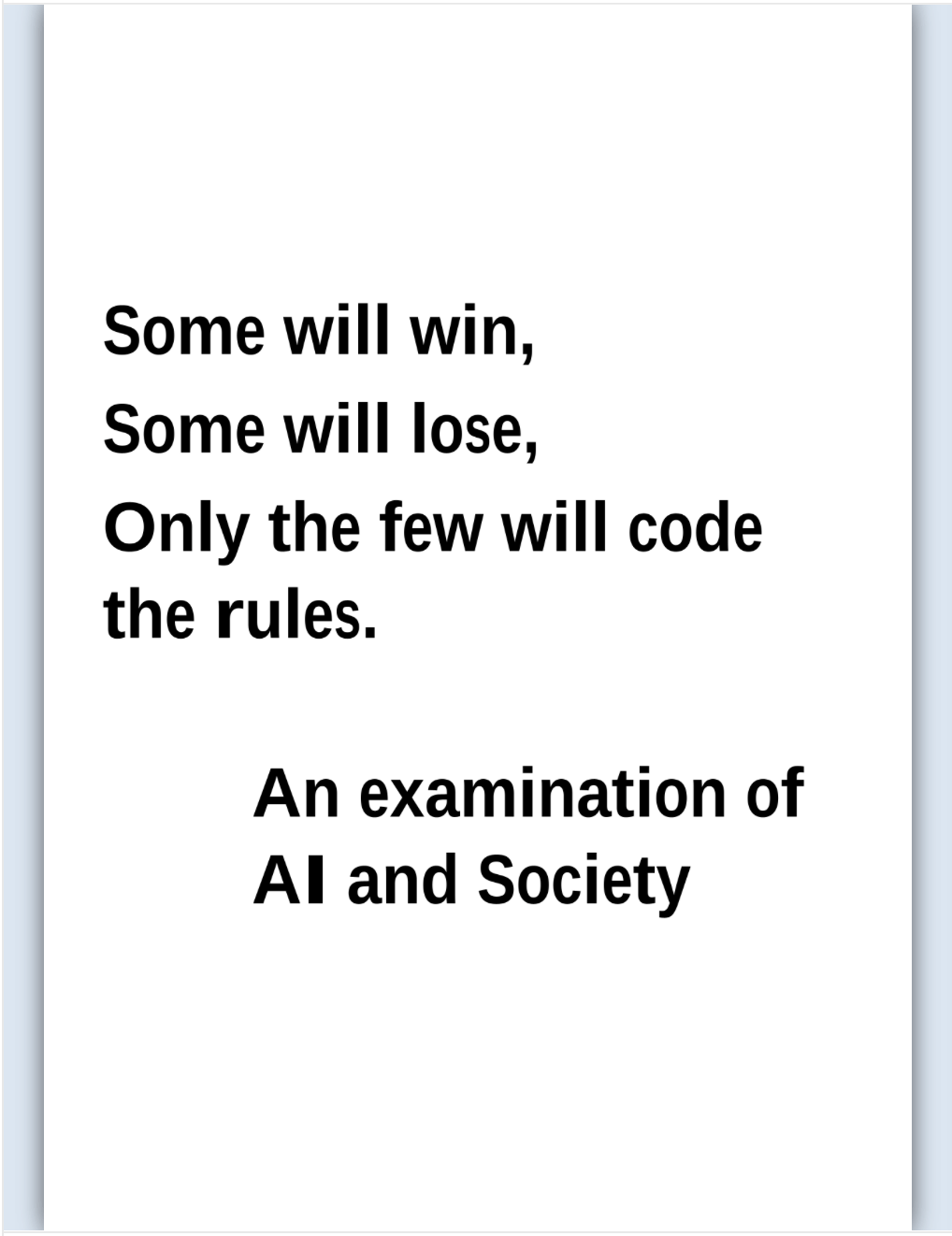
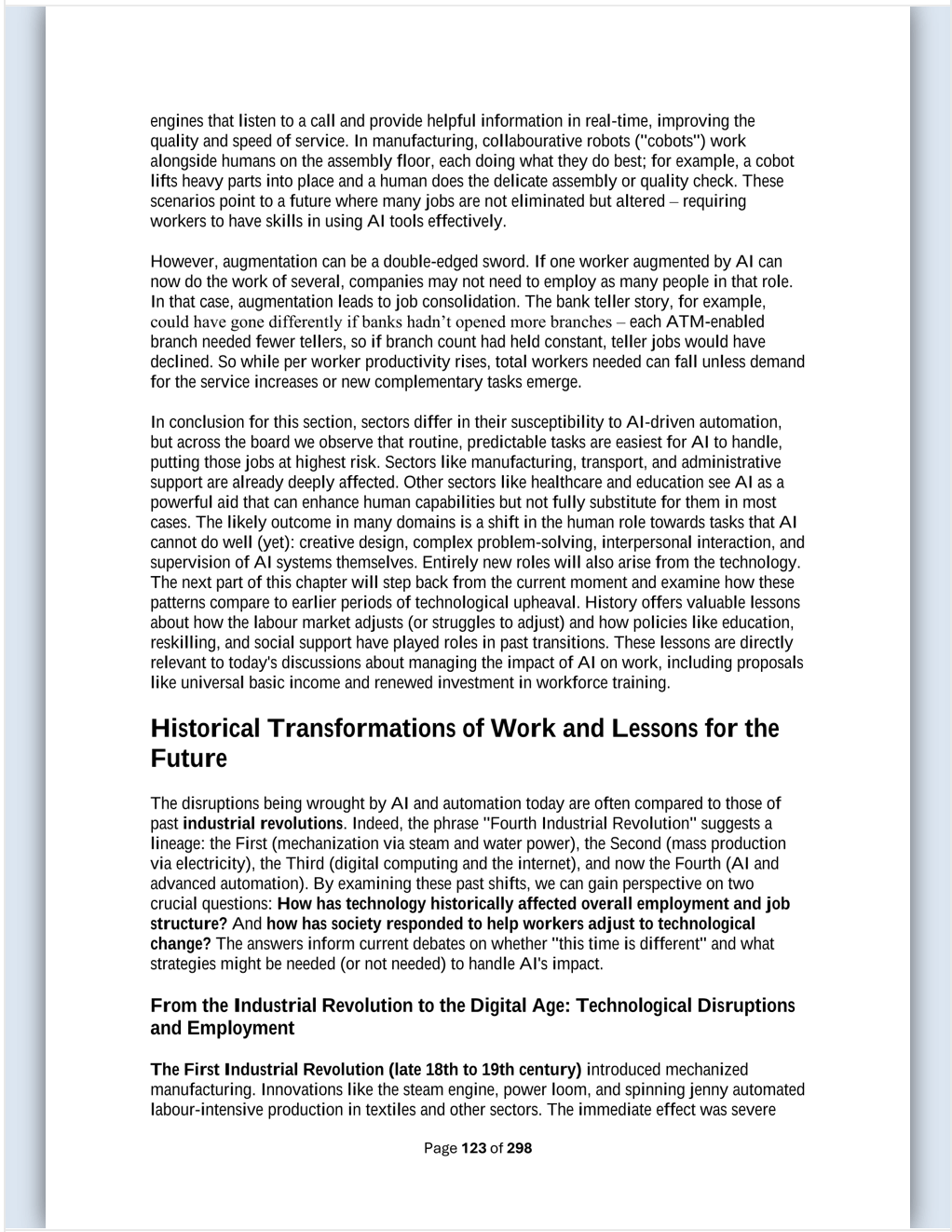
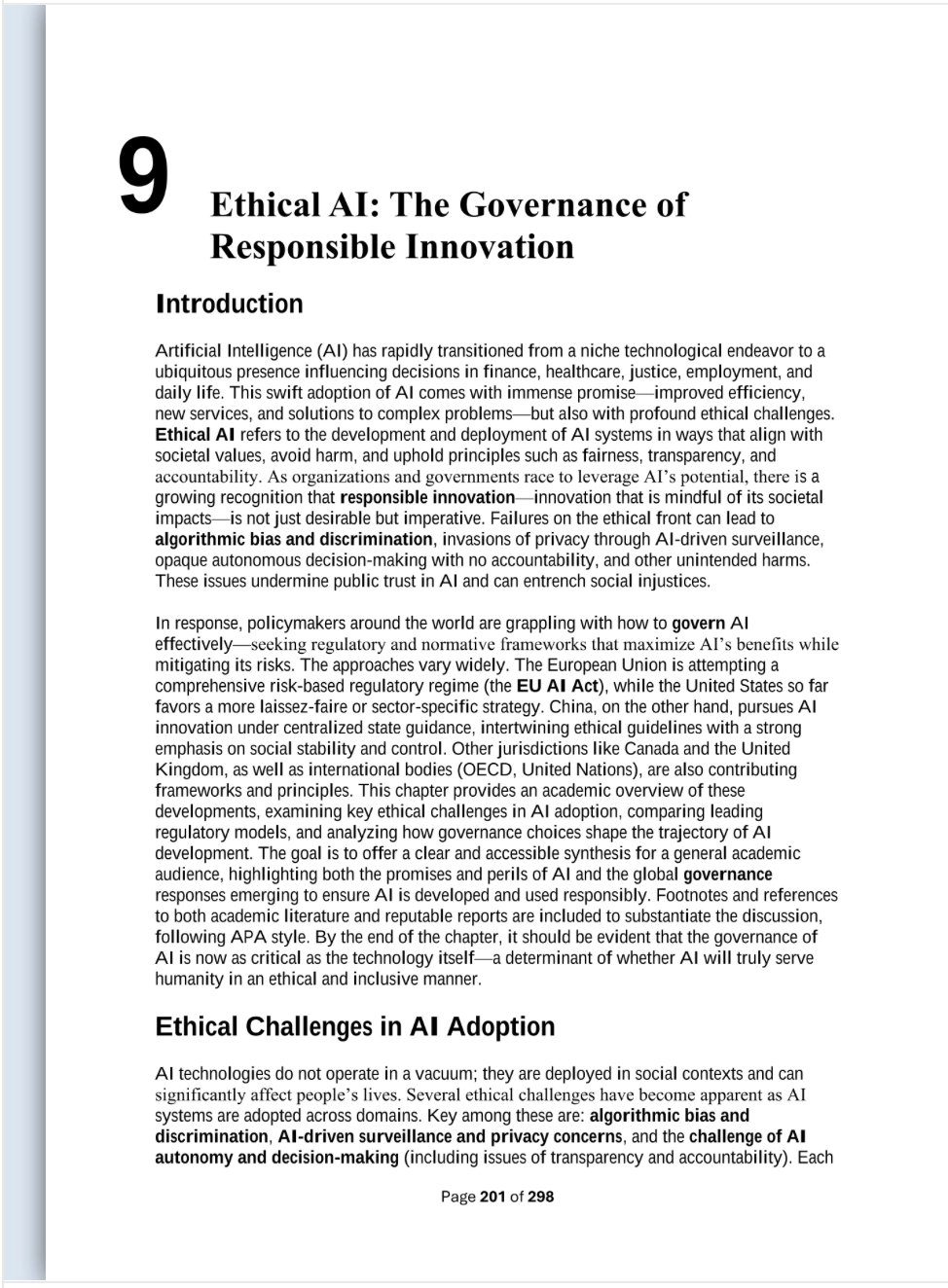
My colleague Richard used the latest ChatGPT models to create a book. First the outline, then section by section, then asking it to review and improve its own text, adding citations and proper referencing, etc. He’s now getting a team of postdocs to factcheck it and so far they haven’t found anything noteworthy.
Before this he used a much earlier model to create a PhD in two hours. He said that it wouldn’t have been a good PhD by any means, but it did resemble a PhD. But all of this, and I’m sure he won’t mind me saying this, is amateur hour compared to what’s coming.
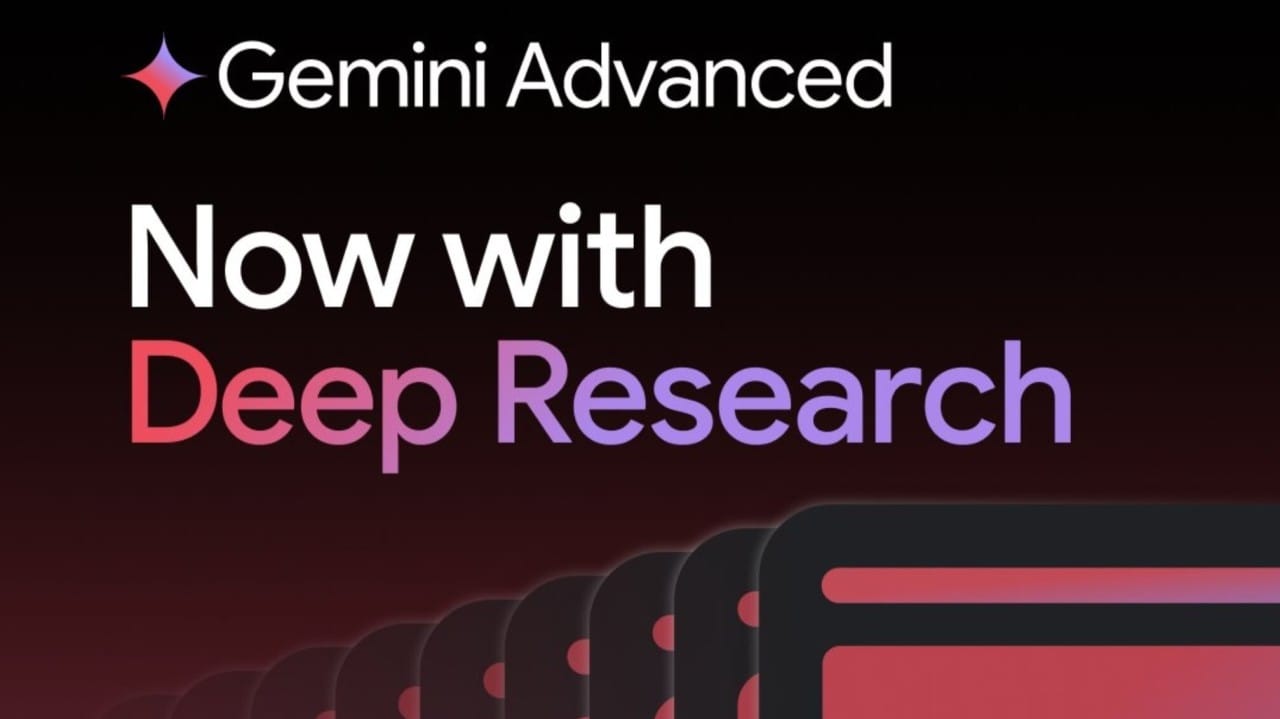
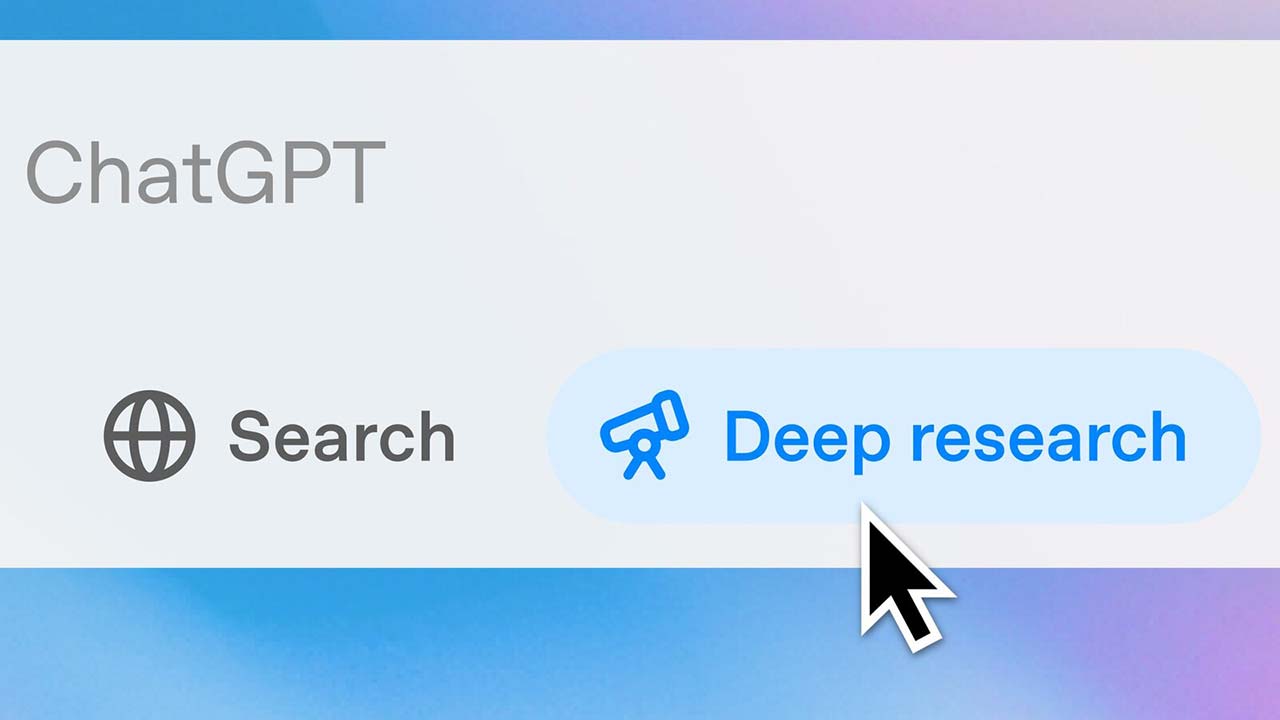
An important development in the past few months has been the public release of research tools that combine the large language models like ChatGPT that we’re familiar with, with Agents that go out and autonomously plan and act and do things online, and with Reasoning, so they can take time to “think”.
We have as examples Google’s Gemini Deep Research, ChatGPT Deep Research and - you’ll detect a pattern here – Perplexity Deep Research (powered by DeepSeek). One that’s bucking the trend is Elon Musk’s tool Grok which is called Deep Search.
I would have been satisfied to see something like it from a beginning PhD student.
Ethan Mollick
I would equate the output with what a strategy consulting team could produce in two or three days working flat out.
Azeem Azhar
These tools will take a question that you provide, ask some clarifying questions and then go off and spend 10 minutes searching the internet, asking itself more questions, doing some more searching, pulling together all of the sources and writing a fairly analytical report.
Here are a couple of people who are fairly prominent commentators on AI giving their reviews on Open AI’s Deep Research. Just remember that anyone can access this tool for less than £20 a month.
Context windows
Another development is increased context windows. So the context window is how much material that you can feed into the large language model to get a response. You can, particularly if you pay for the upgraded models, feed in several books and a whole collection of articles and ask specific questions of them. This can be a huge timesaver for a researcher.
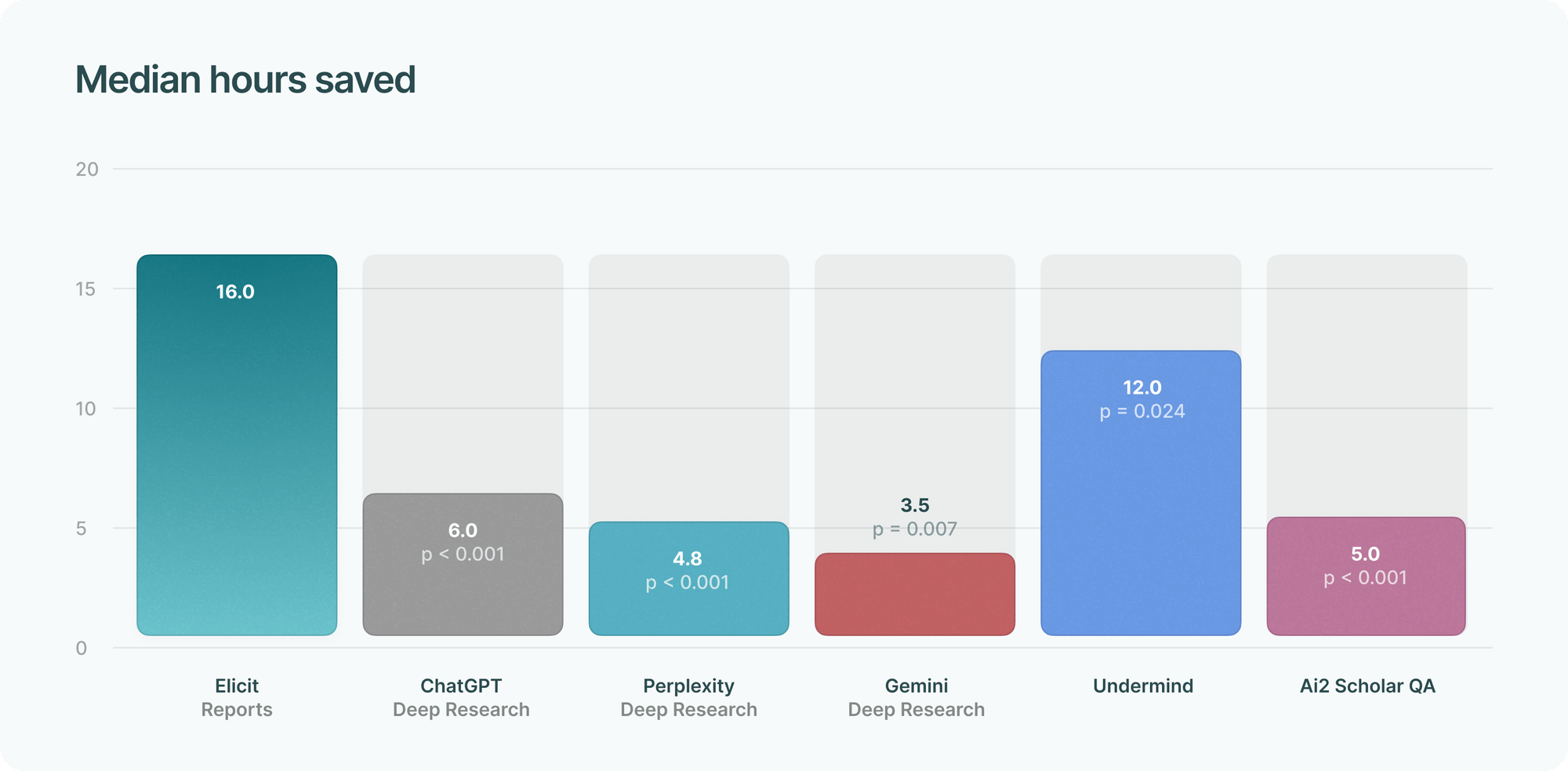
We also have specialised tools that claim to be able to conduct systematic reviews. I have found these quite helpful for sourcing new material, but they are often limited to stuff that isn’t behind paywall so they can only read the abstracts. But they are very good at pulling together a lot of sources and providing quite targeted answers to specific questions.
They tend to perform better for questions demanding answers on randomised control trials and similar studies, and so can favour STEM subjects and economics.
This is the results of a study conducted by Elicit. This is a tool for AI literature reviews and they asked 17 PhD holders how much time each one of these tools would save them when conducting a systematic review. You can see on average they found Elicit would save them 16 hours.
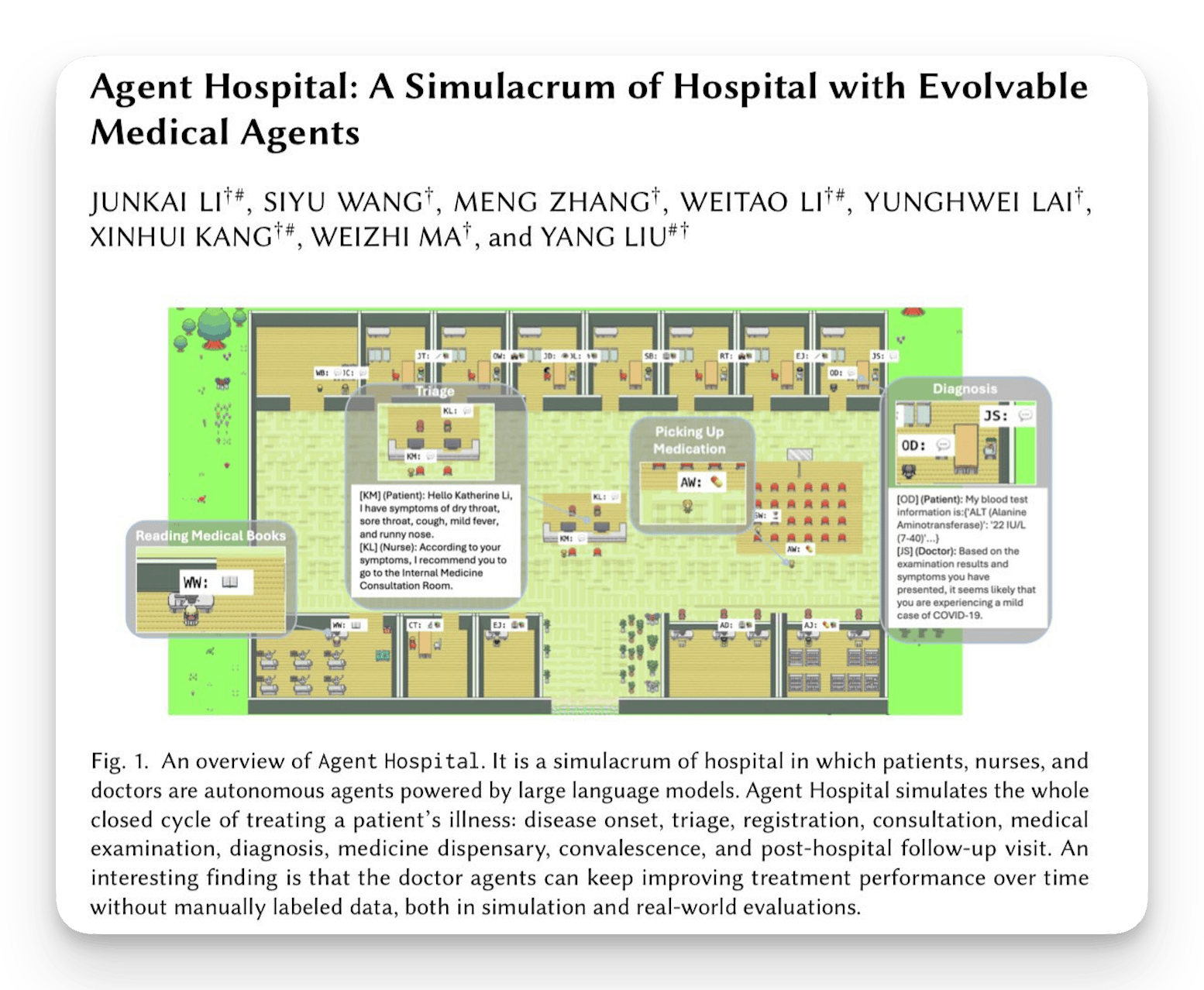
And there’s interesting stuff going on in the social sciences as well. Now, AI can, to a limited extent, simulate human beings, and so you can reproduce famous experiments using AI.
There’s also been some studies where they’ve simulated doctors in a simulated hospital with simulated patients learning how to better diagnose diseases. There’s a lot that we may be able to learn, but obviously AI agents are not humans, so we have to interpret these very carefully.
The democratisation of research
And this is pretty much where we are at the moment. Tools do not replace systematic literature reviews or experiments conducted by experts, but they can speed things up.
This reflects the broader state of AI for research. There are potentially significant time savings for researchers and this offers some democratisation. So with some money, around £20 per month for an top model, some technical ability, being able to use these models effectively and know what to ask, and, most importantly, some contextual knowledge to evaluate the output, AI can sharpen the performance and efficiency of researchers.
At the same time, we are not yet replacing researchers.
Where we might be in 24 months
For the next two years, I will focus on two elements. The first is the use of Agents and the second is how AI may affect the productivity and output of individuals in universities.
An agent is an autonomous intelligent entity capable of performing appropriate and contextually relevant actions.
And just to clarify, here’s what we mean by an agent. They can go off and do things. They can operate in a physical or virtual environment, or a mix of the two.
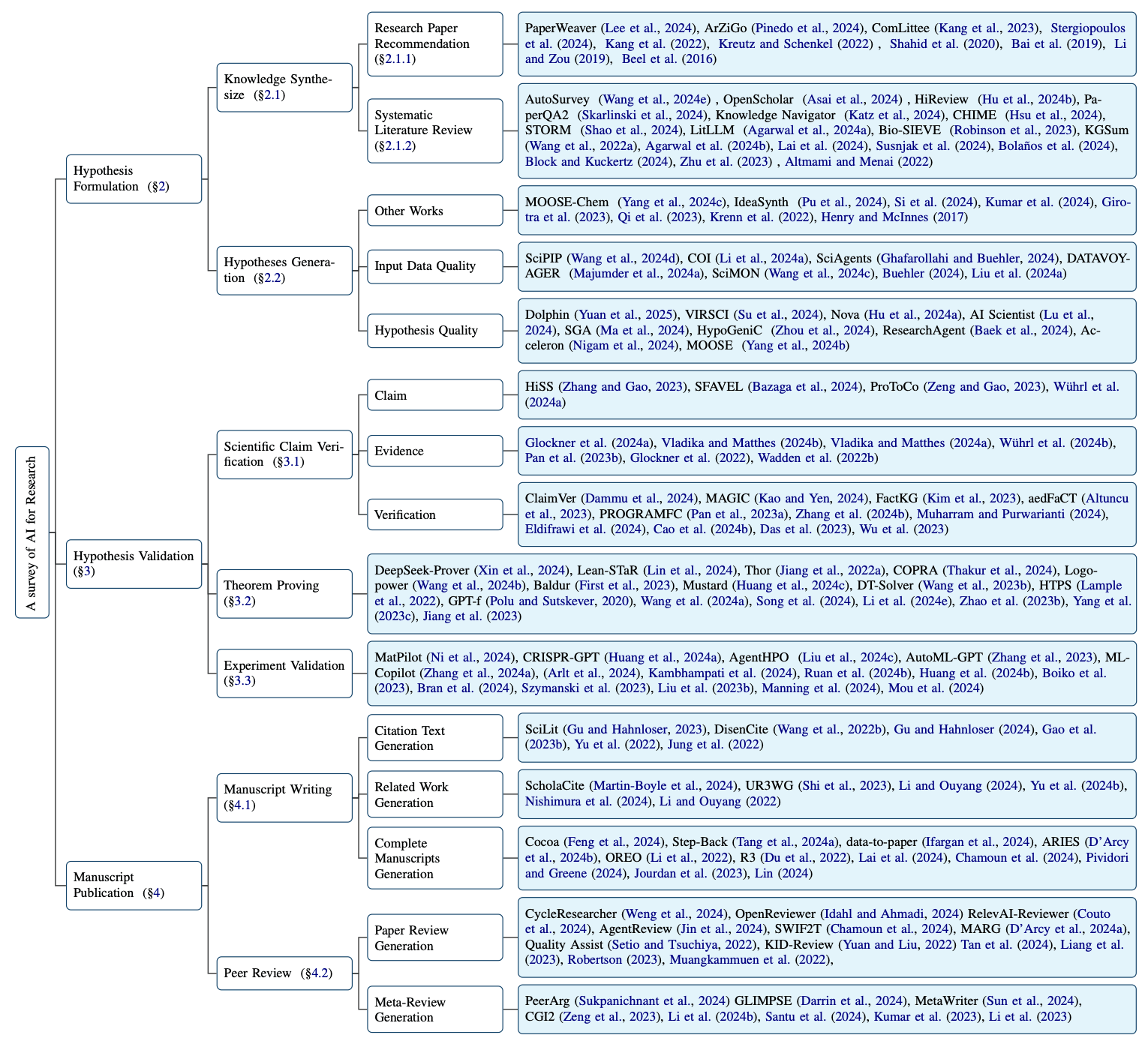
We’re currently seeing a flurry of prototypes of agents that are designed to automate parts of the research process. There’s been work to understand and chop up the research process, automate it and then try and stitch it all together so one AI agent or a series of AI agents can conduct end-to-end research, from generating ideas to publishing papers.
Over the next two years we’ll see development, refinement and increased sophistication of these agents.
See for example this taxonomy of hypothesis formulation, hypothesis validation and manuscript publication, and then different tools for every part.
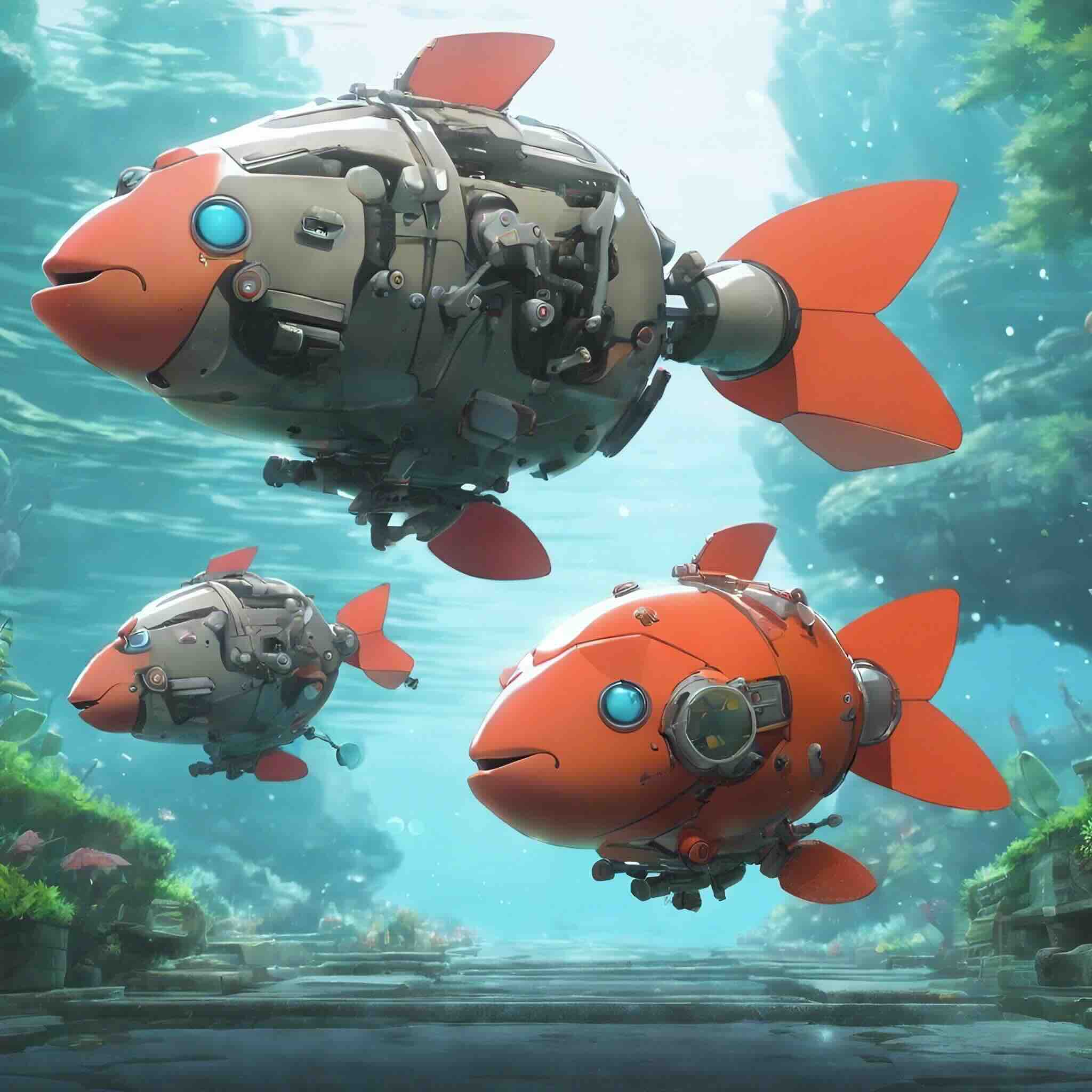
And now two prototype AI research examples. The first is called the ‘AI Scientist’ created by academics at the University of Oxford and the University of British Columbia and a company called Sakana AI based in Japan (Sakana is fish in Japanese).
“it has been common for AI researchers to joke amongst themselves that “now all we need to do is figure out how to make the AI write the papers for us!” Our work demonstrates this idea has gone from a fantastical joke so unrealistic everyone thought it was funny to something that is currently possible.”
Here’s what the creators said in their launch blog post.
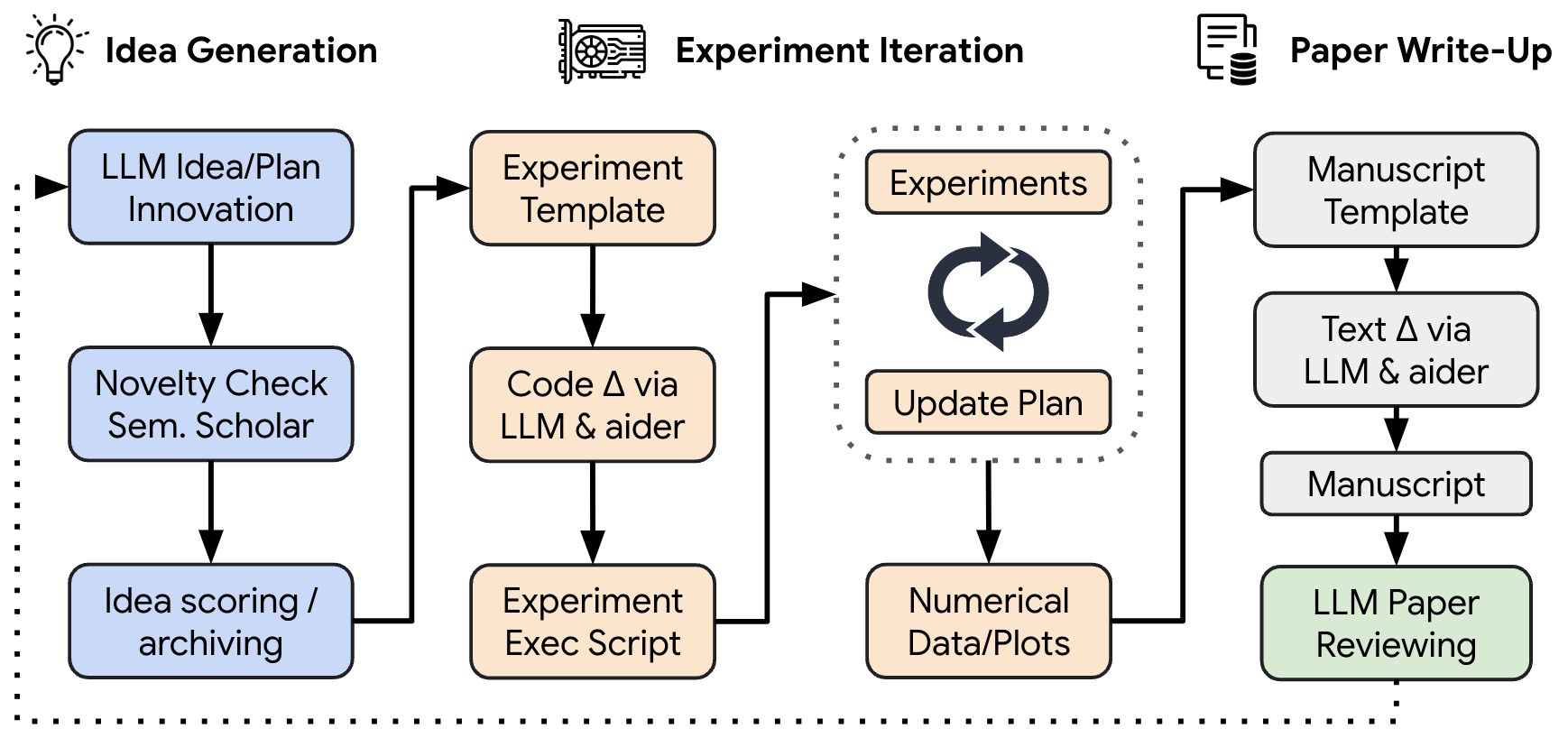
The AI Scientist generates research ideas, writes code, executes experiments, visualises results, describes its findings by writing a full scientific paper and then runs a review process for evaluation. The cost of this is less than $15 per paper.
This diagram shows the end-to-end scientific discovery process.
And they’ve tested it: the agent created a paper from scratch, submitted it to a peer reviewed conference and it beat the acceptance threshold, ahead of many human entries. The organisers knew of this test but the reviewers didn’t.
Interestingly the paper that was submitted reported a negative result which is an important component of effective scientific progress. They then withdrew the paper.
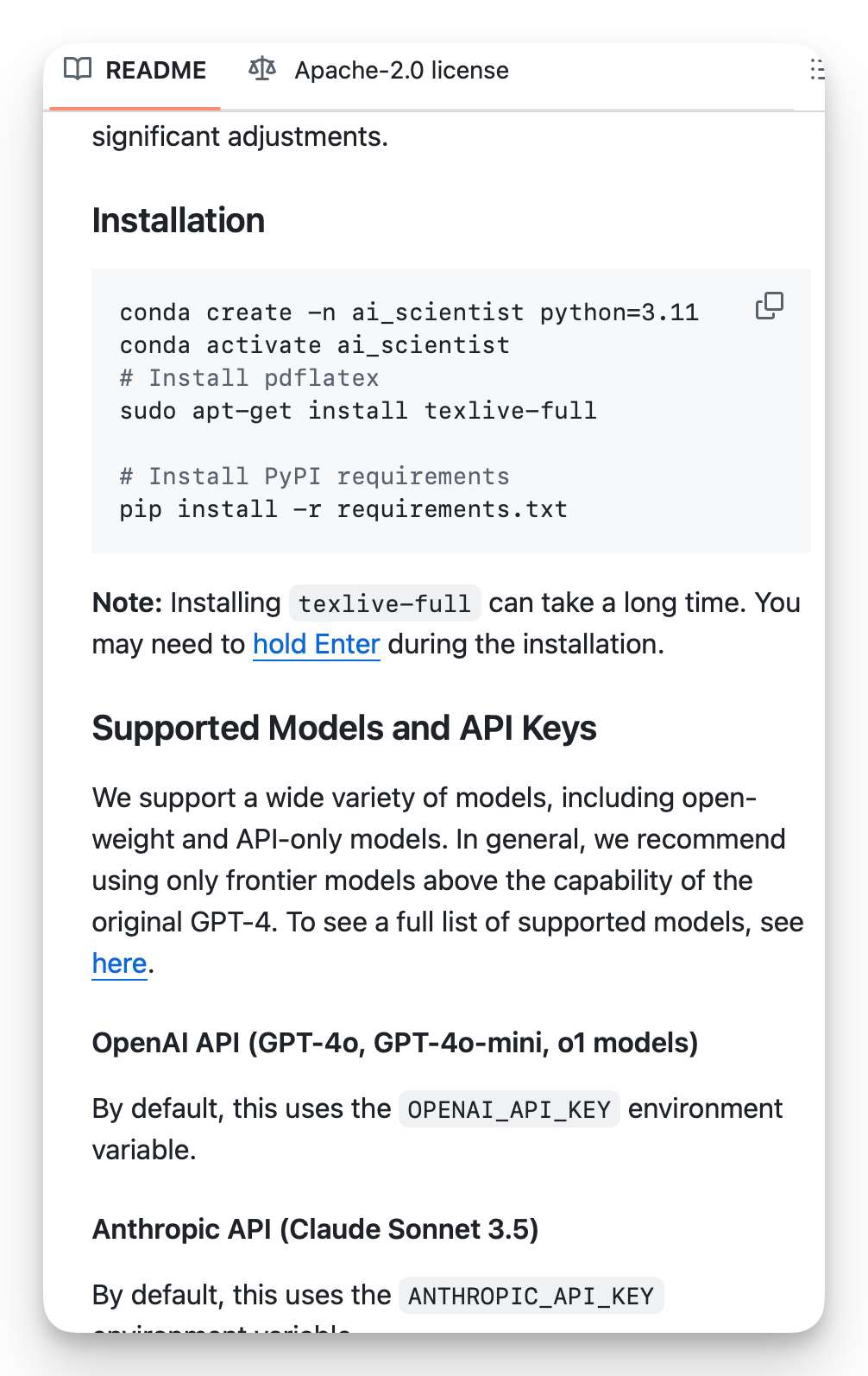
The code for the AI Scientist is open source and anyone can install it on their computer (if their computer is powerful enough).
There are a few shortcomings which the creators reckon they’ll fix quite soon, including generating some tables that don’t fit on pages and not having vision capabilities so it can’t fix things like graphs that are not very readable.
There are also some ethical issues that the creators highlight, including the potential to create an overwhelming number of papers and overload publishing systems, and unintended harms. These harms are particularly around biological materials and so on, if later iterations are given access to laboratories and can conduct actual experiments, or the creation of computer viruses.
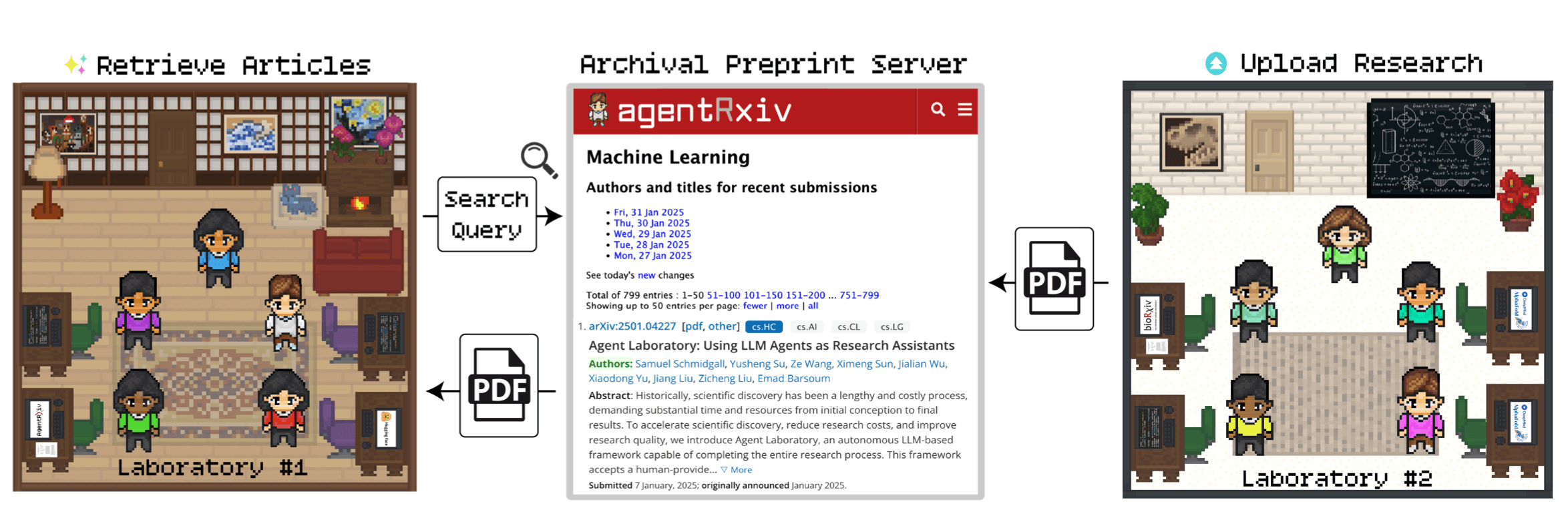
The second example is the AgentRxiv Framework for Autonomous Research Collaboration. This builds on things like the AI Scientist and imagines a future with many AI laboratories. This enables them to feed in their findings to a central repository and learn from each other, enabling AI laboratories to build on each other’s findings and in theory to accelerate scientific progress.
This mirrors the scientific discovery process where work is often the product of many individual researchers or groups of researchers incrementally building on each other’s work, or combining previous ideas. The creators found agents that have access to the archive achieve higher performance compared to those working in isolation.
“we do not believe that the role of a human scientist will be diminished. If anything, the role of a scientist will change and adapt to new technology, and move up the food chain.”
What do these agents mean for researchers in the next few years? The creators of the AI Scientist say this…
What this might mean…
In reality, I suspect that some researchers will greatly benefit. Think of the Pareto principle where a minority of researchers tend to have the greatest number of citations, impact, etc. These sort of power relationships are likely to be enhanced by AI with some researchers disproportionately benefiting (and not necessarily those who are currently in the top 20%).
The vast majority of researchers are probably not going to move up the food chain. But I think there’s other impacts of AI that will benefit researchers in the short term.
This includes using AI to enhance communication. Reframing research to make it relevant to different stakeholder groups is something that can be done easily with AI. Similarly, it can help promote interdisciplinarity by making research from other fields more accessible.
Over the next couple of years AI will do increasingly more of the ‘heavy lifting’ of research tasks, under the expert guidance of academics. We’ll see more disclosure statements in papers along the lines of “this section was assisted by AI”.
Issues like AI-generated misinformation making it into academic work could surface – say an AI misquotes a source and a researcher doesn’t catch it, leading to a retraction or correction. Such incidents will be learning experiences that tighten protocols.
Also the avalanche of papers that we saw at the start is going to continue, although a a great and growing number of these are going to be AI created. AI might become an intermediary, or a layer, between all the research work and the researchers themselves. This brings challenges and opportunities. And we’ll pick up some of these in the next and final section.
Where we might be in 5 years
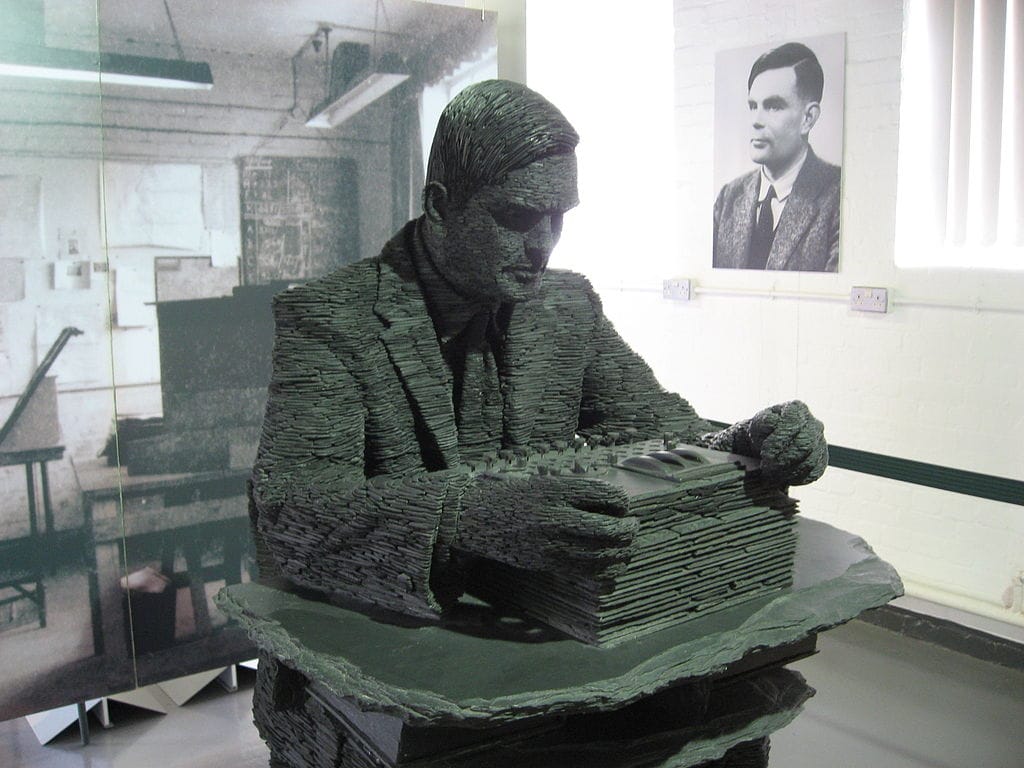
The Turing AI scientist grand challenge
The Alan Turing Institute, which is the UK’s National Centre for Data Science and artificial intelligence, set up an ‘AI scientist grand challenge’ in 2020. It calls for the creation of an AI system capable of making Nobel quality scientific discoveries in an autonomous way by the year 2050.
If some AI researchers are to be believed, we have a very good chance of completing this grand challenge well before 2050.
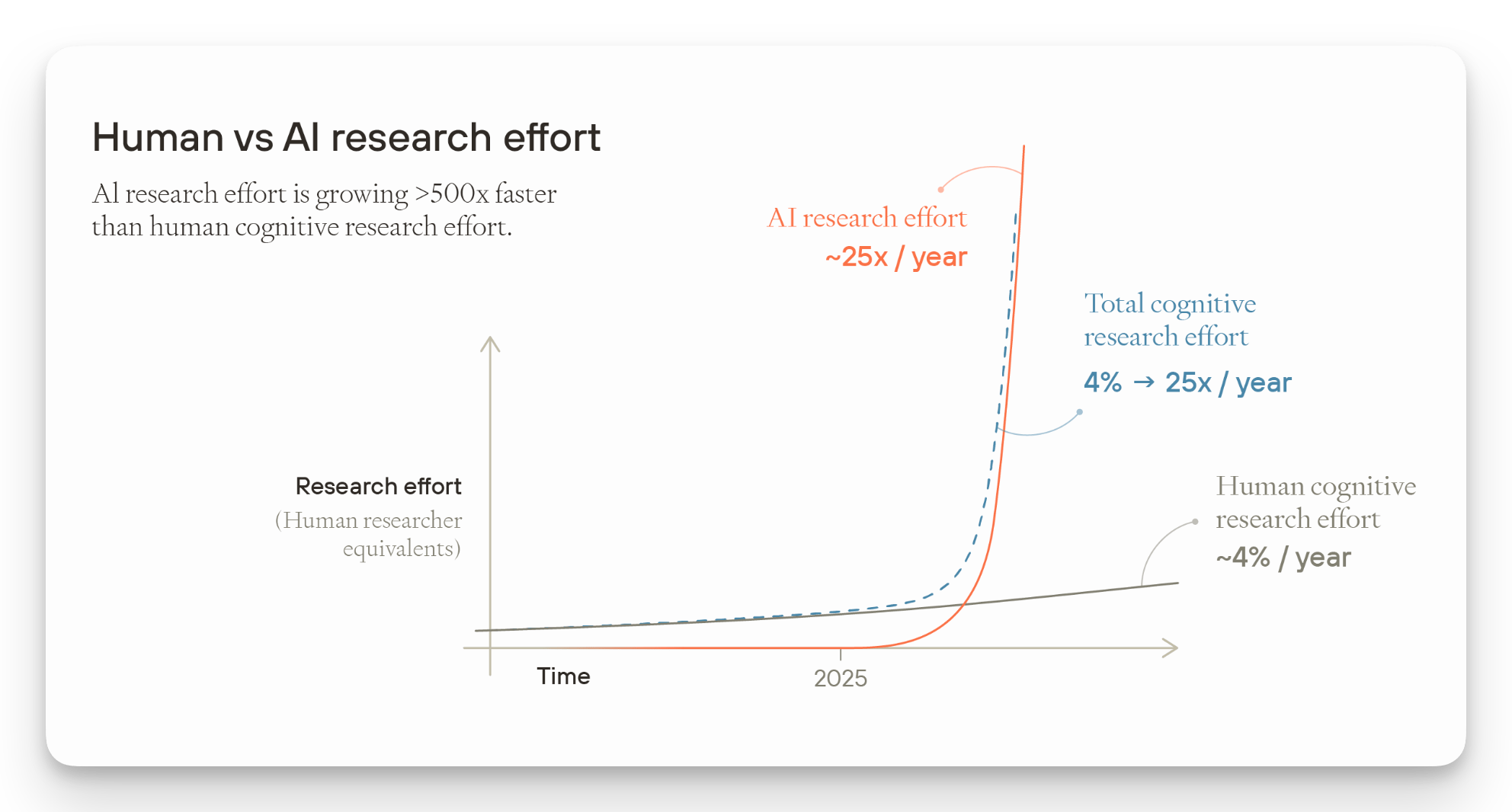
Take for example a report called ‘Preparing for the Intelligence Explosion’ published just a couple of weeks ago by Finn Moorhouse and William MacAskill who are two respected researchers in the field; MacAskill is also a professor of philosophy at Oxford and one of the leading figures behind the ‘effective altruism’ movement.
They make the point that AI cognitive labour, as they call it, is growing more than 500x faster than total human cognitive labour. They say that the amount of research that AI can do is still well below the amount coming from humans. But if it continues growing at its current rate it’ll soon overtake humans. See this graph, with its familiar shape.
They make the point that progress will be different across various subjects, so those that lend themselves more to theoretical reasoning may progress faster than those that require expensive or slow experiments.
And as AI becomes smarter it will be able to train in turn smarter AI systems, in theory driving an intelligence explosion.
Potential scenario driven by advanced AI
A century of technological progress within the next 10 years
The paper is quite detailed and covers a lot of the caveats, different types of diminishing returns, the likelihood of progress being sustained, and the different components of this, and so on.
They have fairly complex modelling where they land on a ‘conservative scenario’, suggesting we might see a century of technological progress within the next 10 years. Let that sink in for a second.
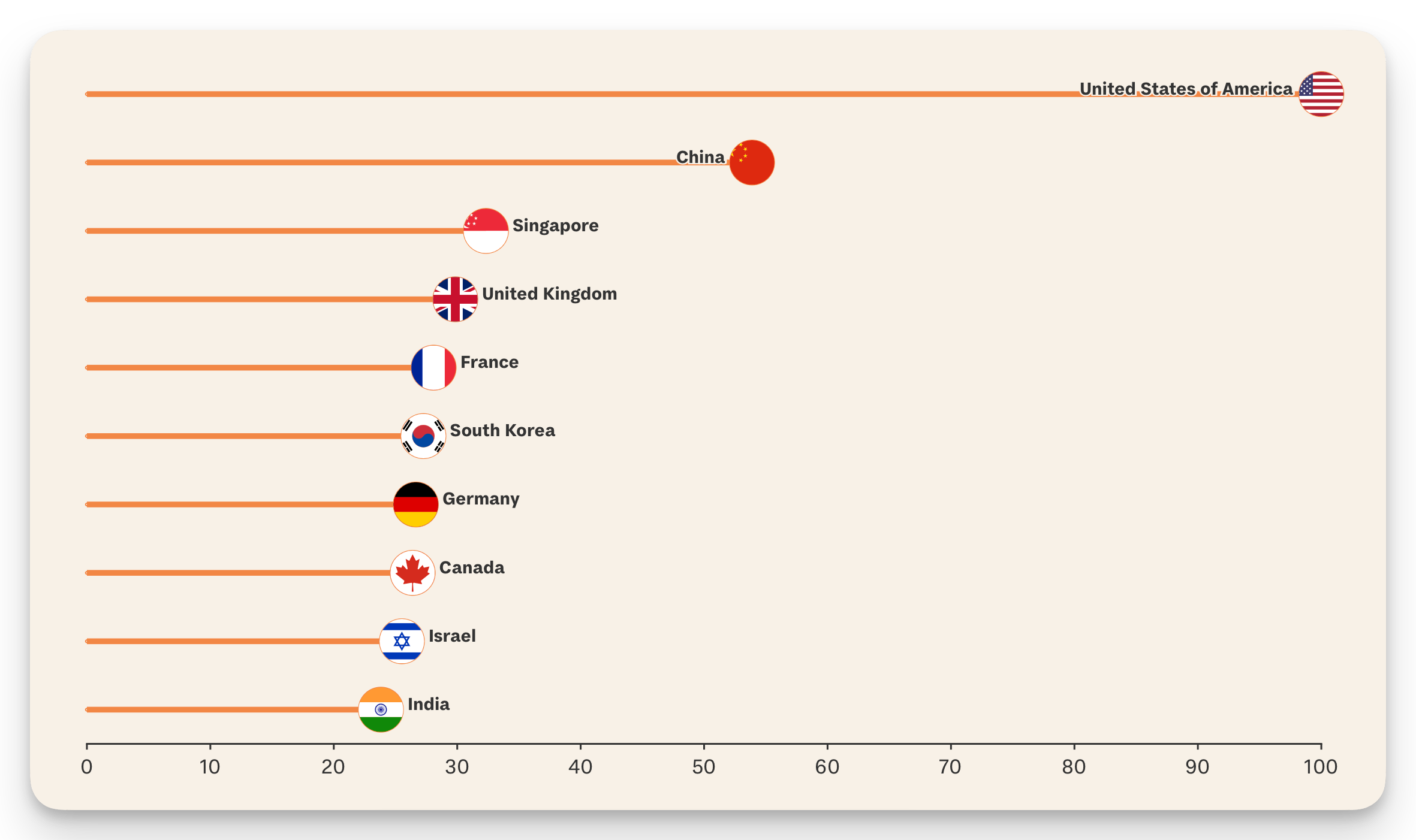
For my final few comments I will take a step back. As I said at the start, we really don’t know whether this is actually going to happen or whether we might see a lesser variation of this. But I think it’s really important that universities take a moment to think: if some variation of this does happen, what it might mean for you?
Above all, if it happens, is this ‘intelligence explosion’ likely to take place in universities, or will it be centralised in and controlled by the large AI labs, who own all the chips?
The centre of power, the investment, the talent is not in universities. All of these things are mostly centred in the US and to a lesser extent China, not in our financially struggling universities (in the UK at least – if you’re in a wealthy university please post your experience in the chat!).
See here analysis by Tortoise media that looks at investment, innovation, and implementation of AI and you can see the US way out in front, followed by China, and the UK in fourth place.
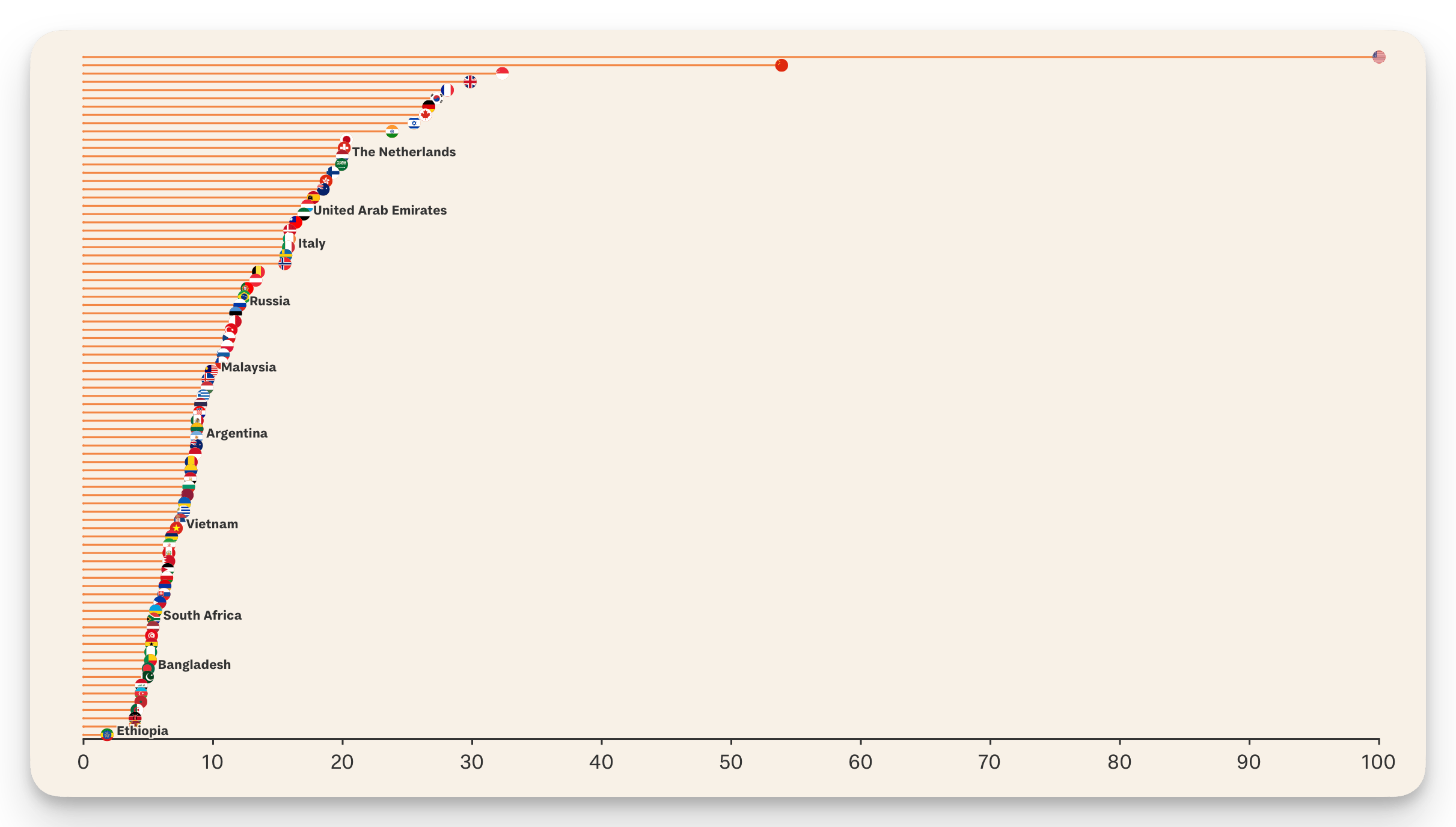
The UK has an AI Opportunities Action Plan which looks to increase UK computer capacity by at least 20x by 2030 and there are some AI by supercomputers in Bristol, Cambridge and Edinburgh, but we’re still a long way behind the US and China. (Apologies non-UK audiences – again, I’m keen to pick up your perspectives in the discussion).
And just to cheer everyone up in the UK, France’s biggest supercomputer is three times more powerful than the UK’s equivalent. Much of the UK’s expertise and leadership in AI has been through Google DeepMind, which is an American-owned company.
This zoomed out graph of all 73 countries included in the analysis shows how far ahead the US is (but I’m guessing China will close the gap a little in the next version).
What this might mean: ownership and risks
So there are some risks we have to consider here. The first is that this activity, as things stand, is likely to be owned by AI labs and not universities. Big tech owns the platforms. As a result there’s a risk of over-concentration of power in tech-funded research and the interests of those companies.
There may be blind spots in the AI systems, including biases in the training data or favouring AI capabilities – so focusing on research where AI tends to do better and neglecting those where it’s not as competent.
This may or may not map on to some of those domains where AI is being used more. So for example STEM versus arts, humanities and social sciences – if it can’t be completely automated, it won’t accelerate. As a result there may be more research done in areas where the AI is capable as it’s cheaper than hiring humans.
Then there are associated risks with highly competent AI about giving access to laboratories to conduct live experiments – and we’re already seeing some fully automated labs, although these are rare at the moment.
And then the need for top grade security to prevent hacking of the systems, and so on. This is a whole separate discussion!
What this might mean: the separation of researchers and universities?
My best guess at how this will play out: the brightest, most successful academics, who are also technically literate and understand AI, will be fine. They will oversee hybrid AI-human teams in research labs, across both STEM and non-STEM, but this will be highly uneven across disciplines. Researchers who don’t fit this category, at financially struggling institutions, are in trouble.
It also points to a future where universities, at least based on the UK model, are no longer such important centres of research. Instead we need to look at the separation between key researchers and universities.
One can imagine a future where a few researchers with prestige, expertise and broad contextual knowledge direct research projects at, or funded by, AI labs and in turn add legitimacy and a human face to the research output.
How would this work in practice? Would universities be compensated? Would research funding agencies still fund projects within universities or would they fund individuals to conduct that research however is most effective?
Is the future university one that conducts research, or one that analyses and translates it into practice, acting as a bridge to society? This is a topic we will be exploring more in the final session.
Key takeaway
Universities should prepare for the possibility that the way research is conducted is going to undergo a seismic shift.
I will finish where we started: with the key takeaway.
Discussion
[email protected]
linkedin.com/in/ransomjames
Thanks for listening (or reading this!); please keep in contact.
Cover image credit: Zoya Yasmine / Better Images of AI / The Two Cultures / CC-BY 4.0